このページは、nAGライブラリのJupyterノートブックExampleの日本語翻訳版です。オリジナルのノートブックはインタラクティブに操作することができます。
%%javascript
.OutputArea.prototype._should_scroll = function(lines) {
IPythonreturn false;
}
このノートブックは、nAGポスターnAGライブラリにおける最近接相関行列に示されているデータを作成しました。
from numpy import genfromtxt,floor,ceil,transpose,nan,zeros,isnan,mean,sum,diag,sqrt,eye,ones
from naginterfaces.library.correg import corrmat_nearest,corrmat_target,corrmat_fixed,corrmat_nearest_bounded
from naginterfaces.library.correg import corrmat_h_weight,corrmat_shrinking,corrmat_nearest_rank
from naginterfaces.base.utils import NagValueError
from numpy.random import rand,seed
from numpy.linalg import eig,norm
import matplotlib.pyplot as plt
import matplotlib
from pytictoc import TicToc
import numpy as np
import pandas as pd
import timeit
import warnings
"ignore",category=matplotlib.cbook.mplDeprecation)
warnings.filterwarnings(
def cor_bar(P):
"""
cor_bar Calculates approximate sample correlation matrix.
S=COR_BAR(P)
Produces an n-by-n approx correlation matrix based on
data of size m-by-n. n columns of different
random variables observed at m different times.
P has missing data represented by NaNs.
INPUT: P data matrix
OUTPUT: R approx sample correlation matrix
"""
= P.shape
(m,n) = cov_bar(P)
S = diag(1./sqrt(diag(S)))
D
= D@S@D
R return(R)
def cov_bar(P):
"""
cov_bar Calculates approximate sample covariance matrix.
S = cov_bar(P)
Produces an n-by-n approx covariance matrix based on
data of size m-by-n. n columns of different
random variables observed at m different times.
P has missing data represented by NaNs.
INPUT: P data matrix
OUTPUT: S approx sample covariance matrix
"""
= P.shape
(m,n)
= zeros((n,n));
S
for i in range(n):
= P[:,i]
xi
for j in range(i+1):
= P[:,j]
xj
# データ値が「一般的」であるマスクを作成する
= ~isnan(xi) & ~isnan(xj)
p
= transpose((xi[p] - mean(xi[p]))) @ ( xj[p] - mean(xj[p]))
S[i,j]
# 有効サンプルサイズで正規化する、つまり sum(p)-1
= 1.0/(sum(p)-1)*S[i,j]
S[i,j] = S[i,j];
S[j,i]
return(S)
def plot_matdiff(x,g,maxdiff,xlabel,funcname,filename=False):
# G と X の差を各要素について小さな塗りつぶされた四角形としてプロットする
= plt.subplots(figsize=(14, 7))
fig1, ax1 = ax1.imshow(abs(x - g), interpolation='none', cmap=plt.cm.Blues,
cax1 =0, vmax=0.2)
vmin= fig1.colorbar(cax1, ticks = np.linspace(0.0, maxdiff, 11, endpoint=True),
cbar =np.linspace(0.0, maxdiff, 11, endpoint=True))
boundaries0, maxdiff])
cbar.set_clim([='both', which='both',
ax1.tick_params(axis='off', top='off', left='off', right='off',
bottom='off', labelleft='off')
labelbottomr'$|G-X|$ for {0}'.format(funcname))
ax1.set_title(
plt.xlabel(xlabel)if filename:
plt.savefig(filename)
plt.show()
def gen_all_ncm_data(file,percent=0.1,n=6,random_seed=2,verbose=False):
"""
Generates random data to benchmark NCM routines
Firstly a fixed block case G = [A B; B^Y C] where
A should be preserved. (Tests TWO and THREE)
We also use this matrix to benchmark without
weighting or fixing. (ONE)
Next we fix or weight C also. (FOUR and FIVE)
THREE and FIVE also set a minimum eigenvalue.
file - Filename of input data. Should be a .csv
percent (Default=0.1) - Fraction of observations removed #TODO - CHANGE TO MORE DESCRIPTIVE VARIABLE
n (Default = 6) - Size of NCM matrix to consider = n x n
"""
seed(random_seed)= genfromtxt(file, delimiter=',')
full_data # 最初の列には行タイトルがあった場所にNaNが含まれているため、これらを削除する必要があります。
= full_data[:,1:]
full_data
# データセット全体から最初のn行だけを抽出する
= full_data[:n,:]
data = data.shape
(n,obvs)
# 固定ブロックのサイズ
= int(floor(n/2))
k # 欠損値の数
= percent * obvs * (n-k)
missing
if verbose:
print('Test matrix size is {0}x{0}'.format(n))
# 1 固定ブロック
= transpose(data.copy())
data1
# k+1:n 変数から「欠損」要素を削除する
= obvs * (n-k)
elements for j in range(int(missing)):
= ceil(rand()*elements)
pos int(pos % obvs),int(k+ceil(pos/obvs)-1)] = nan
data1[
# 近似相関行列を構築する
= cor_bar(data1)
g1
if verbose:
# g1の最小固有値を求めます。負の値になるはずです。
# もし負でなければ、それはすでに有効な相関行列であり、何もする必要はありません
= eig( g1[:n,:n] )
(vals,vecs) = min( vals )
eig_n print('Smallest Eigenvalue of g1 should be negative and it is {0}'.format(eig_n))
# 2 固定ブロック
= transpose(data.copy())
data2
# 対角ブロックを良好に保ち、非対角を悪くするために行を移動する
= int(ceil(percent*obvs))
num_rows = nan
data2[:num_rows,:k] -num_rows:obvs,k:n] = nan
data2[obvs
= cor_bar(data2)
g2
return(g1,g2)
def test_corrmat_nearest(g,num_repeats,plot=True,usemaxscale=False,setmaxscale=0.001,plotfilename=False,verbose=True):
= TicToc()
t
t.tic()for repeat in range(num_repeats):
= corrmat_nearest(g)
(X_g02aa, itera, feval, nrmgrd) = t.tocvalue() / num_repeats
time = norm( X_g02aa-g, 'fro' )
res if verbose:
print('corrmat_nearest (g02aa) time={0} its={1}, norm={2}'.format(time, itera, res))
if plot:
= setmaxscale
maxscale if usemaxscale:
= np.max(abs(X_g02aa - g))
maxscale = "Iterations: {0}, $||G-X||_F = {1:.4f}$".format(itera,norm(X_g02aa - g))
xlabel 'corrmat_nearest',plotfilename)
plot_matdiff(X_g02aa,g,maxscale,xlabel,return(time,res)
def test_corrmat_fixed(g,alpha,h,m,num_repeats,plot=True,usemaxscale=False,setmaxscale=0.001,plotfilename=False,verbose=True):
= h.astype(int)
h = TicToc()
t
t.tic()for repeat in range(num_repeats):
try:
= corrmat_fixed(g,alpha,h,m,errtol=0.0, maxit=100)
(X_g02as,itera,fnorm) = t.tocvalue() / num_repeats
time except NagValueError:
print('corrmat_fixed failed to converge. Returning nan as the time.')
= nan
time return((time,nan))
= t.tocvalue() / num_repeats
time = norm( X_g02as-g, 'fro' )
res if verbose:
print('corrmat_fixed (g02as) time={0} its={1}, norm={2}'.format(time, itera, res))
if plot:
= setmaxscale
maxscale if usemaxscale:
= np.max(abs(X_g02as-g))
maxscale = "Iterations: {0}, $||G-X||_F = {1:.4f}$".format(itera,norm(X_g02as - g))
xlabel 'corrmat_fixed',plotfilename)
plot_matdiff(X_g02as,g,maxscale,xlabel,return(time,res)
def test_corrmat_h_weight(g,alpha,h,num_repeats,plot=True,usemaxscale=False,setmaxscale=0.001,plotfilename=False,verbose=True):
= TicToc()
t
t.tic()for repeat in range(num_repeats):
= corrmat_h_weight(g,alpha,h,maxit=int(500))
(X_g02aj,itera,norma) = t.tocvalue() / num_repeats
time = norm( X_g02aj-g, 'fro' )
res if verbose:
print('corrmat_h_weight (g02aj) time={0} its={1}, norm={2}'.format(time, itera, res))
if plot:
= setmaxscale
maxscale if usemaxscale:
= np.max(abs(X_g02aj-g))
maxscale = "Iterations: {0}, $||G-X||_F = {1:.4f}$".format(itera,norm(X_g02aj - g))
xlabel 'corrmat_h_weight',plotfilename)
plot_matdiff(X_g02aj,g,maxscale,xlabel,return(time,res)
def test_corrmat_target(g, theta, h,num_repeats,plot=True,usemaxscale=False,setmaxscale=0.001,plotfilename=False,verbose=True):
= TicToc()
t
t.tic()for repeat in range(num_repeats):
= corrmat_target(g, theta, h, errtol=0.0, eigtol=0.0)
(h,X_g02ap,alpha,itera,eigmin,norma) = t.tocvalue() / num_repeats
time = norm( X_g02ap-g, 'fro' )
res if verbose:
print('corrmat_target (g02ap) time={0} its={1}, norm={2}'.format(time, itera, res))
if plot:
= setmaxscale
maxscale if usemaxscale:
= np.max(abs(X_g02ap-g))
maxscale = "Iterations: {0}, $||G-X||_F = {1:.4f}$".format(itera,norm(X_g02ap - g))
xlabel 'corrmat_target',plotfilename)
plot_matdiff(X_g02ap,g,maxscale,xlabel,return(time,res)
def test_corrmat_nearest_bounded(g,opt,alpha,w,num_repeats,plot=True,usemaxscale=False,setmaxscale=0.001,plotfilename=False,verbose=True):
= TicToc()
t
t.tic()for repeat in range(num_repeats):
= corrmat_nearest_bounded(g,opt,alpha,w)
(X_g02ab,itera,feval,nrmgrd) = t.tocvalue() / num_repeats
time = norm( X_g02ab-g, 'fro' )
res if verbose:
print('corrmatt_nearest_bounded (g02ab) time={0} its={1}, norm={2}'.format(time, itera, res))
if plot:
= setmaxscale
maxscale if usemaxscale:
= np.max(abs(X_g02ab-g))
maxscale = "Iterations: {0}, $||G-X||_F = {1:.4f}$".format(itera,res)
xlabel 'corrmat_nearest_bounded',plotfilename)
plot_matdiff(X_g02ab,g,maxscale,xlabel,return(time,res)
def test_corrmat_shrinking(g,k,num_repeats,plot=True,usemaxscale=False,setmaxscale=0.001,plotfilename=False,verbose=True):
= TicToc()
t
t.tic()for repeat in range(num_repeats):
= corrmat_shrinking(g,k)
(X_g02an,alpha_out,itera,eigmin,norma) = t.tocvalue() / num_repeats
time = norm( X_g02an-g, 'fro' )
res if verbose:
print('corrmat_shrinking (g02an) time={0} its={1}, norm={2}'.format(time, itera, res))
if plot:
= setmaxscale
maxscale if usemaxscale:
= np.max(abs(X_g02an-g))
maxscale = "Iterations: {0}, $||G-X||_F = {1:.4f}$".format(itera,res)
xlabel 'corrmat_shrinking',plotfilename)
plot_matdiff(X_g02an,g,maxscale,xlabel,return(time,res)
def test1(g,repeats=10,plot=True,verbose=True):
"""
Computes the nearest correlation matrix, all elements in the original matrix are free to move.
"""
= g.shape
(n,m) if verbose:
print('Test 1 - size={0}'.format(n))
print(test1.__doc__)
= 0.0
alpha = 0.0
theta = eye(n)
h
= test_corrmat_nearest(g,num_repeats = repeats,plot=plot,setmaxscale=0.001,plotfilename="test1_corrmat_nearest.png",verbose=verbose)
(nearest_time,nearest_res) = test_corrmat_fixed(g,alpha,h,m=4,num_repeats = repeats,plot=plot,usemaxscale=True,plotfilename="test1_corrmat_fixed.png",verbose=verbose)
(fixed_time,fixed_res) = test_corrmat_target(g,theta, h, num_repeats = repeats,plot=plot,usemaxscale=True,plotfilename="test1_corrmat_target.png",verbose=verbose)
(target_time,target_res)
= pd.DataFrame(np.array([[n,nearest_time,fixed_time,target_time]]))
results =["MatSize","corrmat_nearest","corrmat_fixed","corrmat_target"]
results.columns= results.set_index('MatSize')
results
= pd.DataFrame(np.array([[n,nearest_res,fixed_res,target_res]]))
norms =["MatSize","corrmat_nearest","corrmat_fixed","corrmat_target"]
norms.columns= norms.set_index('MatSize')
norms
return(results,norms)
def test2(g,repeats=10,plot=True,verbose=True):
"""
Computes the nearest correlation matrix, preserving, if possible, an upper left block of the original matrix.
"""
# テスト 2
= g.shape
(n,m) if verbose:
print('\nTest 2 - size={0}'.format(n))
print(test2.__doc__)
= TicToc()
t
= eye(n)
h = int(floor(n/2)) # size of fixed block
k = ones((k,k))
h[:k,:k] = ones(n)
w = 10
w[:k] = 0.0
theta = 0.0
alpha = 'W'
opt
= test_corrmat_nearest_bounded(g,opt,alpha,w, num_repeats = repeats,plot=plot,usemaxscale=True,plotfilename="test2_corrmat_nearest_bounded.png",verbose=verbose)
(nearest_bounded_time,nearest_bounded_res) = test_corrmat_h_weight(g,alpha,h,num_repeats = repeats,plot=plot,usemaxscale=True,plotfilename="test2_corrmat_h_weight.png",verbose=verbose)
(h_weight_time,h_weight_res) = test_corrmat_shrinking(g,k,num_repeats = repeats,plot=plot,usemaxscale=True,plotfilename="test2_corrmat_shrinking.png",verbose=verbose)
(shrinking_time,shrinking_res) = test_corrmat_fixed(g,alpha,h,m=4,num_repeats = repeats,plot=plot,usemaxscale=True,plotfilename="test2_corrmat_fixed.png",verbose=verbose)
(fixed_time,fixed_res)
= pd.DataFrame(np.array([[n,nearest_bounded_time,h_weight_time,shrinking_time,fixed_time]]))
results =["MatSize","corrmat_nearest_bounded","corrmat_h_weight","corrmat_shrinking","corrmat_fixed"]
results.columns= results.set_index('MatSize')
results
= pd.DataFrame(np.array([[n,nearest_bounded_res,h_weight_res,shrinking_res,fixed_res]]))
norms =["MatSize","corrmat_nearest_bounded","corrmat_h_weight","corrmat_shrinking","corrmat_fixed"]
norms.columns= norms.set_index('MatSize')
norms
return(results,norms)
def test3(g,repeats=10,plot=True,verbose=True):
"""
Computes the nearest correlation matrix, preserving, if possible, an upper left block of the original matrix and specifying a minimum eigenvalue for the result.
"""
= g.shape
(n,m) if verbose:
print('\nTest 3 - size={0}'.format(n))
print(test3.__doc__)
= TicToc()
t
= eye(n)
h = int(floor(n/2)) # size of fixed block
k = ones((k,k))
h[:k,:k] = ones(n)
w = 10
w[:k]
# 固定ブロックの最小固有値を求める、正の値であるべき
= eig( g[:k,:k] )
(b1_vals,b1_vecs) = min( b1_vals )
eig_b1
= 0.001
alpha = alpha/eig_b1
theta ='B'
opt
= test_corrmat_nearest_bounded(g,opt,alpha,w,num_repeats = repeats,plot=plot,usemaxscale=True,plotfilename="test3_corrmat_nearest_bounded.png",verbose=verbose)
(nearest_bounded_time,nearest_bounded_res) = test_corrmat_h_weight(g,alpha,h,num_repeats = repeats,plot=plot,usemaxscale=True,plotfilename="test3_corrmat_h_weight.png",verbose=verbose)
(h_weight_time,h_weight_res) = test_corrmat_shrinking(g,k,num_repeats = repeats,plot=plot,usemaxscale=True,plotfilename="test3_corrmat_shrinking.png",verbose=verbose)
(shrinking_time,shrinking_res)= test_corrmat_fixed(g,alpha,h,m=4,num_repeats = repeats,plot=plot,usemaxscale=True,plotfilename="test3_corrmat_fixed.png",verbose=verbose)
(fixed_time,fixed_res)
= pd.DataFrame(np.array([[n,nearest_bounded_time,h_weight_time,shrinking_time,fixed_time]]))
results =["MatSize","corrmat_nearest_bounded","corrmat_h_weight","corrmat_shrinking","corrmat_fixed"]
results.columns= results.set_index('MatSize')
results
= pd.DataFrame(np.array([[n,nearest_bounded_res,h_weight_res,shrinking_res,fixed_res]]))
norms =["MatSize","corrmat_nearest_bounded","corrmat_h_weight","corrmat_shrinking","corrmat_fixed"]
norms.columns= norms.set_index('MatSize')
norms
return(results,norms)
def test4(g,repeats=10,plot = True,verbose=True):
"""
Test 4: Computes the nearest correlation matrix, preserving, if possible, the diagonal blocks of a 2x2 partitioned original matrix.
"""
= g.shape
(n,m) if verbose:
print('\nTest 4 - size={0}'.format(n))
print(test4.__doc__)
= TicToc()
t
= int(floor(n/2)) # size of fixed block
k
= eye(n);
h = ones((k,k))
h[:k,:k] = ones((n-k,n-k))
h[k:n,k:n]
= 0.0
alpha = 0.0
theta
= test_corrmat_h_weight(g,alpha,h,num_repeats = repeats,plot=plot,usemaxscale=True,plotfilename="test4_corrmat_h_weight.png",verbose=verbose)
(h_weight_time,h_weight_res) = test_corrmat_target(g,theta, h, num_repeats = repeats,plot=plot,usemaxscale=True,plotfilename="test4_corrmat_target.png",verbose=verbose)
(target_time,target_res) = test_corrmat_fixed(g,alpha,h,m=4,num_repeats = repeats,plot=plot,usemaxscale=True,plotfilename="test4_corrmat_fixed.png",verbose=verbose)
(fixed_time,fixed_res)
= pd.DataFrame(np.array([[n,h_weight_time,target_time,fixed_time]]))
results =["MatSize","corrmat_h_weight","corrmat_target","corrmat_fixed"]
results.columns= results.set_index('MatSize')
results
def test5(g,repeats=10,verbose=True):
"""
Test 5: Computes the nearest correlation matrix, preserving, if possible, the diagonal blocks of a 2x2 partitioned original matrix and specifying a minimum eigenvalue for the result.
"""
= g.shape
(n,m) if verbose:
print('\nTest 5 - size={0}'.format(n))
print(test5.__doc__)
= TicToc()
t
= int(floor(n/2)) # size of fixed block
k
= eye(n);
h = ones((k,k))
h[:k,:k] = ones((n-k,n-k))
h[k:n,k:n]
# 固定ブロックの最小固有値を求める、正の値であるべき
= eig( g[:k,:k] )
(b1_vals,b1_vecs) = min( b1_vals )
eig_b1 = eig( g[k:n,k:n] )
(b2_vals,b2_vecs) = min( b2_vals )
eig_b2
= 0.001
alpha = min( eig_b1, eig_b2 );
eigmin = alpha/eigmin;
theta ='B'
optif verbose:
print("minimum eigenvalue of the 2 fixed blocks is {0}\n".format(eigmin))
= repeats,plot=True,usemaxscale=True,plotfilename="test5_corrmat_h_weight.png",verbose=verbose)
test_corrmat_h_weight(g,alpha,h,num_repeats = repeats,plot=True,usemaxscale=True,plotfilename="test5_corrmat_target.png",verbose=verbose)
test_corrmat_target(g,theta, h, num_repeats =4,num_repeats = repeats,plot=True,usemaxscale=True,plotfilename="test5_corrmat_fixed.png",verbose=verbose) test_corrmat_fixed(g,alpha,h,m
破損した相関行列の生成
実際の株式データを読み込み、その一部を削除することで破損した相関行列を生成します。ここでは、データの10パーセントを破棄します
= gen_all_ncm_data('data_sp600_06yr.csv',n=200,random_seed=3,percent=0.1,verbose=True) (g1,g2)
Test matrix size is 200x200
Smallest Eigenvalue of g1 should be negative and it is -0.04519164765962588
テスト1
# 最も近い相関行列を計算します。元の行列のすべての要素は自由に動くことができます。
# このプロットは、元の行列と計算された最近接相関行列の差を示しています
# ピクセルが暗いほど、差が大きい
test1(g1)
Test 1 - size=200
Computes the nearest correlation matrix, all elements in the original matrix are free to move.
corrmat_nearest (g02aa) time=0.01880222119999999 its=4, norm=0.19848365625578968
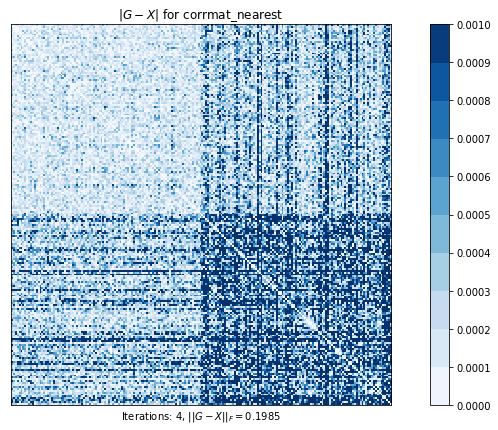
corrmat_fixed (g02as) time=0.12233972249999994 its=32, norm=0.19848383334382902
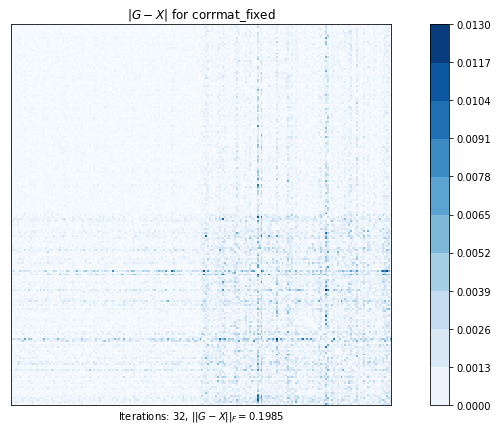
corrmat_target (g02ap) time=0.004642809799999981 its=27, norm=4.974169487825003
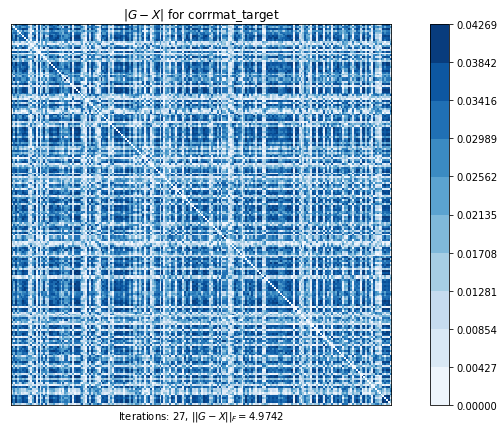
( corrmat_nearest corrmat_fixed corrmat_target
MatSize
200.0 0.018802 0.12234 0.004643,
corrmat_nearest corrmat_fixed corrmat_target
MatSize
200.0 0.198484 0.198484 4.974169)
テスト2
# 可能であれば、元の行列の左上のブロックを保持しながら、最も近い相関行列を計算します。
test2(g1)
Test 2 - size=200
Computes the nearest correlation matrix, preserving, if possible, an upper left block of the original matrix.
corrmatt_nearest_bounded (g02ab) time=0.023739818800000024 its=6, norm=0.2741223031394272
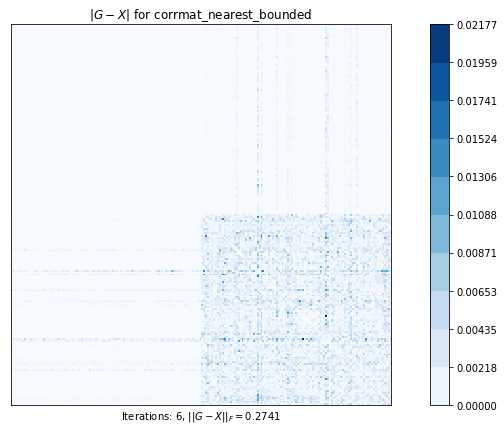
corrmat_h_weight (g02aj) time=0.32874805880000013 its=143, norm=0.2026134982193631
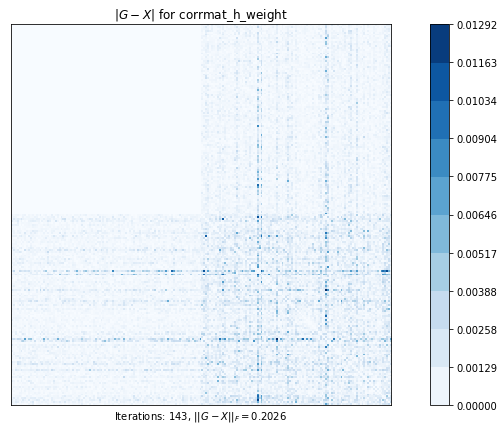
corrmat_shrinking (g02an) time=0.003360725599999981 its=27, norm=4.749647387489478
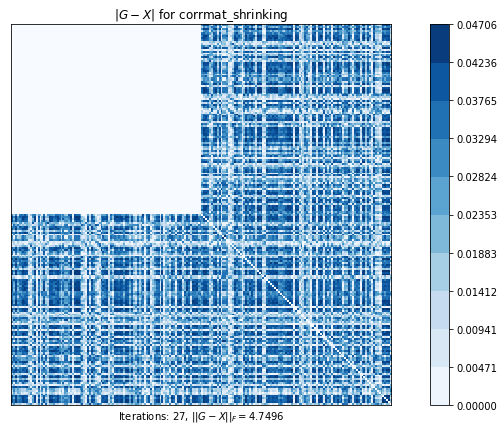
corrmat_fixed (g02as) time=0.12284260710000013 its=32, norm=0.20008340363845287
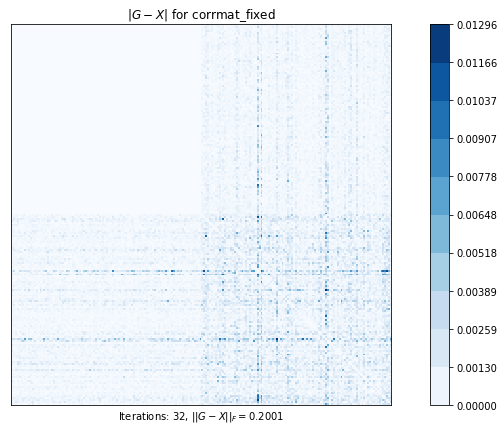
( corrmat_nearest_bounded corrmat_h_weight corrmat_shrinking
MatSize
200.0 0.02374 0.328748 0.003361
corrmat_fixed
MatSize
200.0 0.122843 , corrmat_nearest_bounded corrmat_h_weight
corrmat_shrinking
MatSize
200.0 0.274122 0.202613 4.749647
corrmat_fixed
MatSize
200.0 0.200083 )
テスト3
# 可能な限り保持しながら、最も近い相関行列を計算します。
# 元の行列の左上のブロックを取り出し、結果の最小固有値を指定します。
# このプロットは、元の行列と計算された最近接相関行列の差を示しています
# ピクセルが暗いほど、差が大きい
test3(g1)
テスト3 - サイズ=200
Computes the nearest correlation matrix, preserving, if possible, an upper left block of the original matrix and specifying a minimum eigenvalue for the result.
corrmatt_nearest_bounded (g02ab) time=0.0249553333999998 its=6, norm=0.29188823015833215
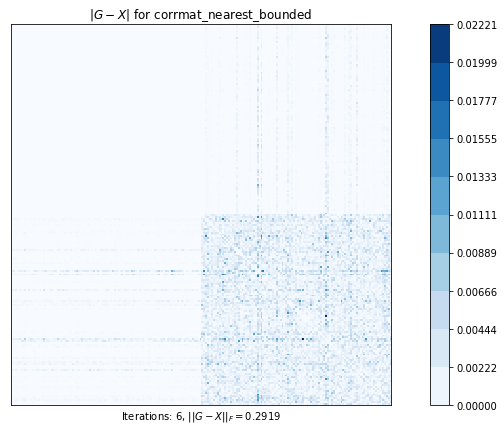
corrmat_h_weight (g02aj) time=0.42672047189999984 its=144, norm=0.21394785831325455
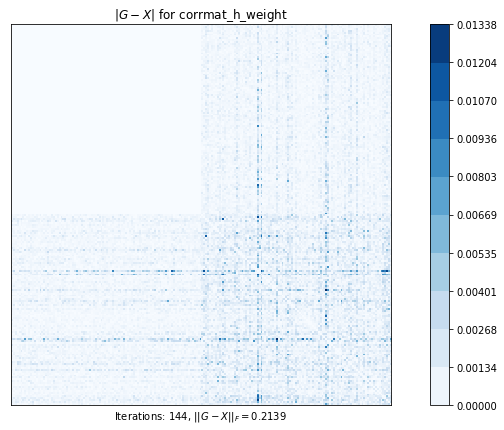
corrmat_shrinking (g02an) time=0.00232538059999996 its=27, norm=4.749647387489478
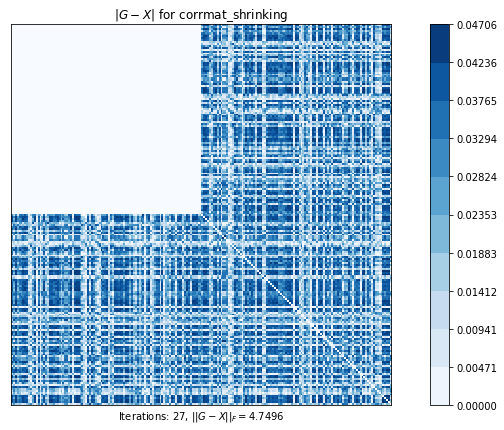
corrmat_fixed (g02as) time=0.14476502070000025 its=40, norm=0.2112270891810479
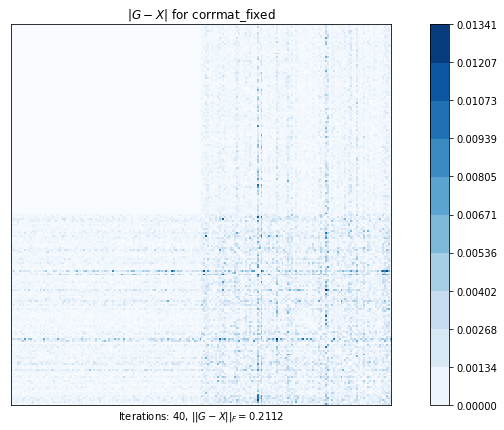
( corrmat_nearest_bounded corrmat_h_weight corrmat_shrinking \
MatSize
200.0 0.024955 0.42672 0.002325
corrmat_fixed
MatSize
200.0 0.144765 ,
corrmat_nearest_bounded corrmat_h_weight corrmat_shrinking \
MatSize
200.0 0.291888 0.213948 4.749647
corrmat_fixed
MatSize
200.0 0.211227 )
テスト4
# 2x2に分割された元の行列の対角ブロックを可能な限り保持しながら、最も近い相関行列を計算します。
# このプロットは、元の行列と計算された最近接相関行列の差を示しています
# ピクセルが暗いほど、差が大きい
test4(g2)
Test 4 - size=200
Test 4: Computes the nearest correlation matrix, preserving, if possible, the diagonal blocks of a 2x2 partitioned original matrix.
corrmat_h_weight (g02aj) time=0.5691674330999998 its=210, norm=4.2415769479815095
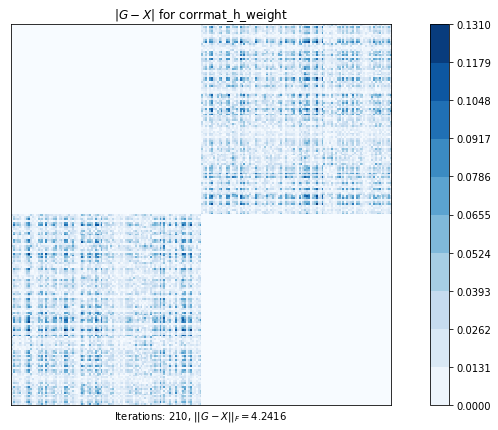
corrmat_target (g02ap) time=0.005968094099999632 its=27, norm=8.216919543483945
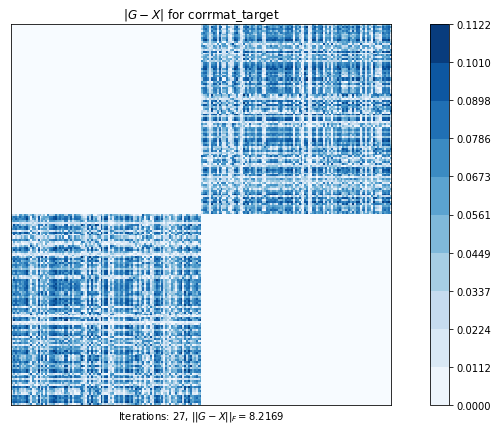
corrmat_fixed (g02as) time=0.09307719119999973 its=25, norm=4.207809588867262
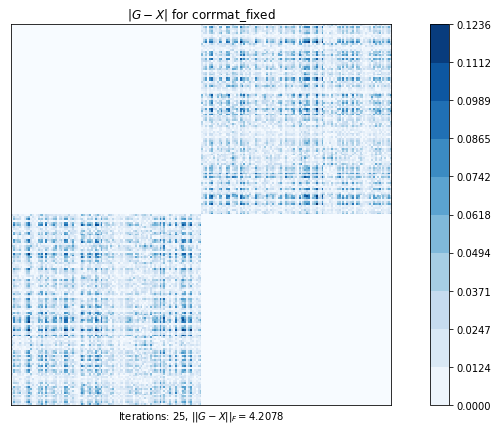
テスト5
# 2x2に分割された元の行列の対角ブロックを可能な限り保持し、結果の最小固有値を指定しながら、最も近い相関行列を計算します。
# このプロットは、元の行列と計算された最近接相関行列との差を示しています
# ピクセルが暗いほど、差が大きい
test5(g2)
Test 5 - size=200
Test 5: Computes the nearest correlation matrix, preserving, if possible, the diagonal blocks of a 2x2 partitioned original matrix and specifying a minimum eigenvalue for the result.
minimum eigenvalue of the 2 fixed blocks is 0.0009119866783221636
corrmat_h_weight (g02aj) time=1.1305440822000001 its=198, norm=4.247239921631062
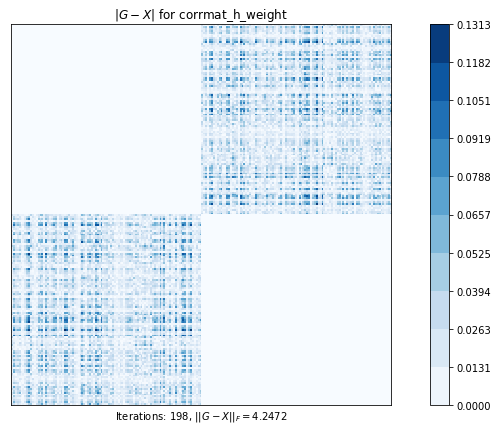
---------------------------------------------------------------------------
NagValueError Traceback (most recent call last)
<ipython-input-8-4b83712cf465> in <module>
2 #The plot shows the difference between the original matrix and the computed Nearest Correlation Matrix
3 #The darker the pixel, the greater the difference
----> 4 test5(g2)
<ipython-input-2-edb83b8903e9> in test5(g, repeats, verbose)
424
425 test_corrmat_h_weight(g,alpha,h,num_repeats = repeats,plot=True,usemaxscale=True,plotfilename="test5_corrmat_h_weight.png",verbose=verbose)
--> 426 test_corrmat_target(g,theta, h, num_repeats = repeats,plot=True,usemaxscale=True,plotfilename="test5_corrmat_target.png",verbose=verbose)
427 test_corrmat_fixed(g,alpha,h,m=4,num_repeats = repeats,plot=True,usemaxscale=True,plotfilename="test5_corrmat_fixed.png",verbose=verbose)
<ipython-input-2-edb83b8903e9> in test_corrmat_target(g, theta, h, num_repeats, plot, usemaxscale, setmaxscale, plotfilename, verbose)
217 t.tic()
218 for repeat in range(num_repeats):
--> 219 (h,X_g02ap,alpha,itera,eigmin,norma) = corrmat_target(g, theta, h, errtol=0.0, eigtol=0.0)
220 time = t.tocvalue() / num_repeats
221 res = norm( X_g02ap-g, 'fro' )
~\AppData\Local\Continuum\anaconda3\envs\nAGdev.105\lib\site-packages\naginterfaces\library\correg.htm in corrmat_target(g, theta, h, errtol, eigtol)
965 errtol,
966 eigtol,
--> 967 _x,
968 )
969 _h_py = _h
~\AppData\Local\Continuum\anaconda3\envs\nAGdev.105\lib\site-packages\naginterfaces\base\correg.htm in corrmat_target(g, n, theta, h, errtol, eigtol, x)
1979 _norm_py,
1980 )
-> 1981 _err_class.manage()
1982 return return_data
1983
~\AppData\Local\Continuum\anaconda3\envs\nAGdev.105\lib\site-packages\naginterfaces\base\utils.htm in manage(self)
750 def manage(self):
751 raise NagValueError(
--> 752 self._set_locus(), _format_msg_lines(self.msg_lines)
753 )
754
NagValueError: (nAG Python function naginterfaces.base.correg.corrmat_target, code 3:3,1003)
** On entry, theta = 0.109650724486432E+01.
** Constraint: theta < 1.0.
スケーリングテスト1
ここでは、様々なソルバールーチンに対して、行列サイズに応じて解決時間がどのように変化するかを確認します。
= range(50,501,50)
sizes = pd.DataFrame()
times = pd.DataFrame()
norms for size in sizes:
= gen_all_ncm_data('data_sp600_06yr.csv',n=size,random_seed=3,percent=0.1,verbose=False)
(g1,g2) = test1(g1,repeats=10,plot=False,verbose=False)
(time,thisnorm) = pd.concat([times,time])
times = pd.concat([norms,thisnorm])
norms 'test1_times.csv')
times.to_csv('test1_norms.csv')
norms.to_csv( times
corrmat_nearest | corrmat_fixed | corrmat_target | |
---|---|---|---|
MatSize | |||
50.0 | 0.001507 | 0.004228 | 0.000743 |
100.0 | 0.005066 | 0.017035 | 0.001638 |
150.0 | 0.010110 | 0.058214 | 0.003499 |
200.0 | 0.016392 | 0.159881 | 0.003662 |
250.0 | 0.024968 | 0.326784 | 0.006156 |
300.0 | 0.035980 | 0.340655 | 0.007679 |
350.0 | 0.060690 | 0.792486 | 0.010839 |
400.0 | 0.076551 | 1.332434 | 0.012364 |
450.0 | 0.082097 | 1.240161 | 0.017019 |
500.0 | 0.103721 | 1.853304 | 0.020717 |
norms
corrmat_nearest | corrmat_fixed | corrmat_target | |
---|---|---|---|
MatSize | |||
50.0 | 0.036431 | 0.036431 | 0.586536 |
100.0 | 0.057950 | 0.057950 | 1.265381 |
150.0 | 0.141608 | 0.141608 | 3.535194 |
200.0 | 0.198484 | 0.198484 | 4.974169 |
250.0 | 0.273451 | 0.273450 | 6.594377 |
300.0 | 0.299582 | 0.299582 | 8.392438 |
350.0 | 0.427399 | 0.427395 | 11.684947 |
400.0 | 0.461658 | 0.461653 | 13.615143 |
450.0 | 0.527523 | 0.527520 | 15.834235 |
500.0 | 0.602729 | 0.602729 | 18.901359 |
# ファイルから読み込んでプロットする
= pd.read_csv('test1_times.csv',index_col=0)
test1_results
test1_results.plot()'Test 1 Time to solution (s)')
plt.title('test1_scaling.png') plt.savefig(
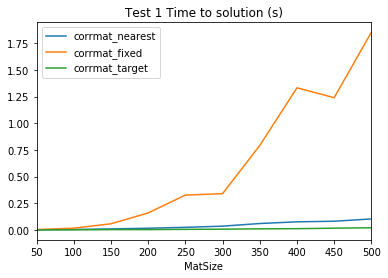
スケーリングテスト 2
= range(50,501,50)
sizes = pd.DataFrame()
times2 = pd.DataFrame()
norms2 for size in sizes:
= gen_all_ncm_data('data_sp600_06yr.csv',n=size,random_seed=3,percent=0.1,verbose=False)
(g1,g2) = test2(g1,repeats=10,plot=False,verbose=False)
(time,thisnorm) = pd.concat([times2,time])
times2 = pd.concat([norms2,thisnorm])
norms2 'test2_times.csv')
times2.to_csv('test2_norms.csv')
norms2.to_csv( times2
corrmat_nearest_bounded | corrmat_h_weight | corrmat_shrinking | corrmat_fixed | |
---|---|---|---|---|
MatSize | ||||
50.0 | 0.002095 | 0.031946 | 0.000545 | 0.004413 |
100.0 | 0.005953 | 0.117579 | 0.000857 | 0.019222 |
150.0 | 0.014070 | 0.265287 | 0.001387 | 0.057748 |
200.0 | 0.021630 | 0.441480 | 0.002309 | 0.158402 |
250.0 | 0.035854 | 0.697917 | 0.003813 | 0.355081 |
300.0 | 0.050336 | 0.980578 | 0.005018 | 0.329038 |
350.0 | 0.067137 | 1.377873 | 0.005678 | 1.153101 |
400.0 | 0.086270 | 1.781721 | 0.006498 | 1.520508 |
450.0 | 0.114125 | 1.899170 | 0.008666 | 1.380511 |
500.0 | 0.145073 | 2.415297 | 0.010312 | 1.982778 |
norms2
corrmat_nearest_bounded | corrmat_h_weight | corrmat_shrinking | corrmat_fixed | |
---|---|---|---|---|
MatSize | ||||
50.0 | 0.061481 | 0.036964 | 0.538910 | 0.036601 |
100.0 | 0.094640 | 0.059782 | 1.317656 | 0.058625 |
150.0 | 0.207350 | 0.144398 | 3.328873 | 0.142627 |
200.0 | 0.274122 | 0.202613 | 4.749647 | 0.200083 |
250.0 | 0.363962 | 0.279006 | 6.315969 | 0.275650 |
300.0 | 0.412061 | 0.305694 | 8.303853 | 0.302068 |
350.0 | 0.544452 | 0.434568 | 11.497122 | 0.430317 |
400.0 | 0.592730 | 0.469202 | 13.413678 | 0.464864 |
450.0 | 0.673075 | 0.537169 | 16.048218 | 0.531557 |
500.0 | 0.756571 | 0.612887 | 19.359882 | 0.607072 |
# ファイルから読み込んでプロット
= pd.read_csv('test2_times.csv',index_col=0)
test2_results
test2_results.plot()'Test 2 Time to solution (s)')
plt.title('test2_scaling.png') plt.savefig(
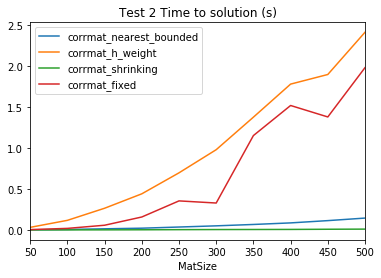
スケーリングテスト 3
= range(50,501,50)
sizes = pd.DataFrame()
times3 = pd.DataFrame()
norms3 for size in sizes:
= gen_all_ncm_data('data_sp600_06yr.csv',n=size,random_seed=3,percent=0.10,verbose=False)
(g1,g2) = test3(g1,repeats=10,plot=False,verbose=False)
(time,thisnorm) = pd.concat([times3,time])
times3 = pd.concat([norms3,thisnorm])
norms3 'test3_times.csv')
times3.to_csv('test3_norms.csv')
norms3.to_csv( times3
corrmat_fixed failed to converge. Returning nan as the time.
corrmat_fixed failed to converge. Returning nan as the time.
corrmat_fixed failed to converge. Returning nan as the time.
corrmat_fixed failed to converge. Returning nan as the time.
corrmat_fixed failed to converge. Returning nan as the time.
corrmat_fixed failed to converge. Returning nan as the time.
corrmat_nearest_bounded | corrmat_h_weight | corrmat_shrinking | corrmat_fixed | |
---|---|---|---|---|
MatSize | ||||
50.0 | 0.002108 | 0.032379 | 0.000552 | 0.004937 |
100.0 | 0.005998 | 0.119652 | 0.000859 | 0.018615 |
150.0 | 0.014243 | 0.275873 | 0.001390 | 0.061095 |
200.0 | 0.021669 | 0.532417 | 0.002306 | 0.198863 |
250.0 | 0.035913 | 1.665548 | 0.003815 | NaN |
300.0 | 0.052591 | 3.065310 | 0.005082 | NaN |
350.0 | 0.068778 | 4.251140 | 0.005748 | NaN |
400.0 | 0.104276 | 5.194119 | 0.006569 | NaN |
450.0 | 0.112667 | 5.857296 | 0.008791 | NaN |
500.0 | 0.143404 | 7.292331 | 0.010422 | NaN |
norms3
corrmat_nearest_bounded | corrmat_h_weight | corrmat_shrinking | corrmat_fixed | |
---|---|---|---|---|
MatSize | ||||
50.0 | 0.066512 | 0.039832 | 0.538910 | 0.039410 |
100.0 | 0.104984 | 0.064710 | 1.317656 | 0.063430 |
150.0 | 0.221349 | 0.152816 | 3.328873 | 0.150886 |
200.0 | 0.291888 | 0.213948 | 4.749647 | 0.211227 |
250.0 | 0.383613 | 0.294094 | 6.315969 | NaN |
300.0 | 0.431817 | 0.319817 | 8.303853 | NaN |
350.0 | 0.566489 | 0.453559 | 11.497122 | NaN |
400.0 | 0.615492 | 0.490537 | 13.413678 | NaN |
450.0 | 0.697933 | 0.561680 | 16.048218 | NaN |
500.0 | 0.781180 | 0.639620 | 19.359882 | NaN |
# ファイルから読み込んでプロットする
= pd.read_csv('test3_times.csv',index_col=0)
test3_results
test3_results.plot()'Test 3 Time to solution (s)');
plt.title('test3_scaling.png') plt.savefig(
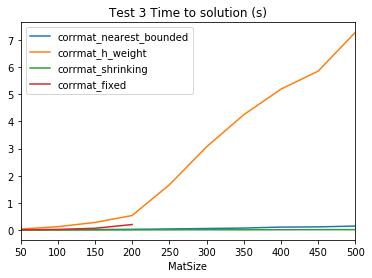
システム情報
スケーリング結果はAmazon EC2インスタンス上で実行されました。システムの詳細はここに示されています
import multiprocessing
!date
!uname -r
!test -d /etc/redhat-release && cat /etc/redhat-release !test -d /etc/debian_version && cat /etc/debian_version !test -d /etc/os-release && cat /etc/os-release
print('CPUS available = {0}'.format(multiprocessing.cpu_count()))
!hostname
!cat /proc/cpuinfo | grep 'model name' | uniq
Tue May 21 09:37:21 UTC 2019
4.14.77-70.59.amzn1.x86_64
CPUS available = 16
ip-172-31-34-138
model name : Intel(R) Xeon(R) CPU E5-2666 v3 @ 2.90GHz