このページは、nAGライブラリのJupyterノートブックExampleの日本語翻訳版です。オリジナルのノートブックはインタラクティブに操作することができます。
非負値行列因子分解を用いたウェブページの分類
自動分類が必要な15のURL
brexit_trump_urls.txt
ファイルには、ニュース記事への15のリンクが含まれています。その中にはドナルド・トランプに関するものと、ブレグジットに関するものがあります。人間であれば読んで簡単に判断できますが、ここではnAG
Library for PythonのNon-negative matrix
factorization関数であるreal_nmfを使用して、これを自動的に行う方法を示します。
# brexit_trump_urls.txtファイル内のURLを表示する
with open('brexit_trump_urls.txt') as f:
= f.read()
read_data print(read_data)
https://www.bbc.co.uk/news/uk-politics-47031312
https://www.bbc.co.uk/news/world-us-canada-47477727
https://www.bbc.co.uk/news/uk-scotland-north-east-orkney-shetland-47642076?intlink_from_url=&link_location=live-reporting-story
https://www.bbc.co.uk/news/uk-wales-politics-47651013?intlink_from_url=https://www.bbc.co.uk/news/topics/cwlw3xz0lvvt/brexit&link_location=live-reporting-story
https://www.bbc.co.uk/news/av/world-us-canada-47646183/president-trump-shows-map-of-is-defeat?intlink_from_url=&link_location=live-reporting-map
https://www.bbc.co.uk/news/uk-politics-46393399
https://www.bbc.co.uk/news/business-47644268?intlink_from_url=&link_location=live-reporting-story
https://www.bbc.co.uk/news/world-us-canada-47633940
https://www.bbc.co.uk/news/uk-politics-47627744
https://www.bbc.co.uk/news/uk-politics-parliaments-47653160
https://www.bbc.co.uk/news/world-us-canada-47642335
https://www.bbc.co.uk/news/uk-politics-47660019
https://www.bbc.co.uk/news/world-middle-east-47657843
https://www.bbc.co.uk/news/uk-politics-47659410
https://www.bbc.co.uk/news/uk-politics-47652071
ウェブサイトの解析
最初のステップは、すべての記事をダウンロードし、それらを単語のセットに解析することです。
from naginterfaces.library.matop import real_nmf
from collections import Counter
import string
import urllib.request
import re
from scipy.linalg import norm
import numpy as np
print("Reading urls")
with open('brexit_trump_urls.txt', 'r') as f:
= f.readlines()
links
= len(links)
n_links
= []
dicts = []
titles = set()
words = str.maketrans(string.punctuation, ' '*len(string.punctuation))
trans
print("Parsing webpages")
for link in links:
= urllib.request.urlopen(link)
f1 = []
pagewords = re.findall(r'<p>(.*?)</p>', f1.read().decode().lower())
paras = urllib.request.urlopen(link)
f2 = re.findall(r'<title data-rh="true">(.*?)</title>', f2.read().decode())
title print(title)
titles.append(title)for para in paras:
+= para.translate(trans).split()
pagewords
= Counter(pagewords)
c
dicts.append(c)= words | set(list(c.keys())) words
Reading urls
Parsing webpages
['Brexit delay: How is Article 50 extended? - BBC News']
['Kirstjen Nielsen: Walking a tightrope working for Trump - BBC News']
['Trump homes plan at Menie being recommended for approval - BBC News']
['Theresa May at her worst during Brexit speech - Mark Drakeford - BBC News']
['President Trump shows map of 'IS defeat' - BBC News']
['Brexit: What happens now? - BBC News']
['Trump spooks markets with China trade tariffs warning - BBC News']
['A tale of two Trumps: Jair Bolsonaro goes to Washington - BBC News']
['Brexit: Theresa May to formally ask for delay - BBC News']
['Corbyn calls for compromise to avoid no-deal Brexit - BBC News']
['Trump: I didn't get a thank you for McCain funeral - BBC News']
['Brexit: EU leaders agree Article 50 delay plan - BBC News']
['Trump: Time to recognise Golan Heights as Israeli territory - BBC News']
['Brexit: MPs urged not to travel home alone as tensions rise - BBC News']
[''Cancel Brexit' petition passes 2m signatures on Parliament site - BBC News']
変数dicts
には15の辞書が含まれており、それぞれがURLごとの単語頻度に対応しています。
以下は最初のURLで最も一般的な単語です
0].most_common(20) dicts[
[(‘the’, 37), (‘a’, 37), (‘to’, 21), (‘uk’, 17), (‘of’, 15), (‘that’, 10), (‘in’, 10), (‘class’, 9), (‘ssrcss’, 9), (‘eu’, 9), (‘it’, 9), (‘on’, 9), (‘bbc’, 9), (‘href’, 8), (‘would’, 8), (‘an’, 7), (‘extension’, 7), (‘and’, 7), (‘www’, 7), (‘co’, 7)]
変数words
には、すべてのURLから見られたすべての単語のリストがアルファベット順で含まれています。
len(words)
2268
単語リストのクリーンアップ
この2268語のリストには、‘the’、‘that’、’with’などの多くの一般的な単語が含まれており、これらは無視したいものです。 これらの不要な単語は一般的にストップワードと呼ばれています。 この分析で使用する具体的なストップワードのリストは、次のセルで定義されています。
= ['then', 'that', 'have', 'with', 'from', 'they', 'here', 'there', 'their', 'would', 'what', 'which', 'about', 'know',
stopwords 'just', 'time', 'like', 'make', 'your', 'year', 'some', 'good', 'into', 'people', 'them', 'other', 'than', 'look',
'only', 'over', 'think', 'also', 'back', 'after', 'work', 'first', 'well', 'even', 'want', 'because', 'these',
'most', 'leave', 'seem', 'come', 'little', 'last', 'long', 'great', 'high', 'small', 'large', 'next', 'early',
'same', 'this', 'away', 'down', 'look', 'make', 'three', 'came', 'four', 'please', 'pretty', 'soon', 'under',
'went', 'white', 'black', 'give', 'giving', 'given', 'gave', 'knowing', 'knew', 'once', 'round', 'stop', 'take',
'taken', 'took', 'thank', 'thanks', 'walk', 'walked', 'always', 'around', 'been', 'before', 'best', 'worst', 'find',
'found', 'goes', 'pull', 'read', 'right', 'wrong', 'tell', 'telling', 'told', 'upon', 'wish', 'write', 'written',
'better', 'carry', 'carried', 'full', 'hold', 'keep', 'kept', 'longer', 'longest', 'shall', 'will', 'begin',
'beginning', 'together', 'today', 'yesterday', 'children', 'ground', 'hold', 'holding', 'morning', 'afternoon',
'never', 'myself', 'table', 'water', 'wind', 'window', 'ring', 'rung', 'except', 'where', 'while', 'woman',
'whilst', 'were', 'until', 'thing', 'things', 'theirs', 'monday', 'tuesday', 'wednesday', 'thursday', 'friday',
'saturday', 'sunday', 'should', 'shown', 'shut', 'another', 'being', 'does', 'doing', 'make', 'makes', 'more',
'name', 'names', 'named', 'through', 'years', 'used', 'said', 'saying', 'says', 'seen', 'sees', 'seeing',
'using', 'uses', 'known', 'left', 'send', 'sent', 'choose', 'choice', 'choosing', 'going', 'gets', 'below',
'less', 'least', 'might', 'href', 'link', 'https', 'a939', 'abbe', 'ac30f6e24b3f', 'ababa']
単語リストからそれらを削除しましょう
= [word for word in words if not word in stopwords] words
また、4文字未満の単語をすべて削除します
= [word for word in words if not len(word)<4] words
len(words)
1873
ここで、数字または#記号で始まる単語を削除します
= [word for word in words if not word.startswith('£')]
words = [word for word in words if not word.startswith('1')]
words = [word for word in words if not word.startswith('2')]
words = [word for word in words if not word.startswith('3')]
words = [word for word in words if not word.startswith('4')]
words = [word for word in words if not word.startswith('5')]
words = [word for word in words if not word.startswith('6')]
words = [word for word in words if not word.startswith('7')]
words = [word for word in words if not word.startswith('8')]
words = [word for word in words if not word.startswith('9')]
words = [word for word in words if not word.startswith('0')] words
print("{0} distinct words will be used to form the data matrix".format(len(words)))
1825個の異なる単語がデータ行列を形成するために使用されます
データ行列の計算
ここで、これらの単語を使用してデータ行列を形成したいと思います。行(i)は単語を、各列(j)はURLを指します。この行列の(i,j)番目のエントリには、URL jにおける単語iの出現頻度が含まれます
# 次に、単語をキーとし、各ウェブページでの単語の出現回数をタプルとする辞書を作成します
= {}
masterdict
print("Creating data matrix")
for word in words:
# 単語数を含むリストを作成する
= [dic.get(word, 0) for dic in dicts]
freqs = freqs
masterdict[word]
= np.asfortranarray(np.array(list(masterdict.values())), dtype=np.float64)
a
print("Final data matrix has size:{0}".format(a.shape))
データ行列の作成 最終的なデータ行列のサイズ:(1825, 15)
a
array([[0., 0., 0., ..., 0., 0., 0.],
[0., 0., 0., ..., 0., 0., 0.],
[0., 0., 0., ..., 0., 1., 0.],
...,
[1., 0., 0., ..., 0., 0., 0.],
[1., 0., 0., ..., 0., 0., 0.],
[0., 0., 0., ..., 0., 0., 0.]])
データ行列は、複数の変数の観測値の集合です。上記の単語カウント行列では、観測値は個々のWebページで、変数はそれらのページ上の異なる単語の頻度でしたが、他にも多くの例があります。例えば、観測値は画像内のピクセルで、変数は分光測定データかもしれません。または、観測値は異なる人々に対応し、変数は様々な検査結果かもしれません。この分析では、データ行列の各列が観測値に対応し、各行が変数に対応すると仮定します。
データ行列には多くの場合、以下のような特性があります。
- エントリーは非負です。これは常にそうとは限りませんが、多くの重要な応用で当てはまります。
- 非常に大きく、疎である可能性があります。最近、地震波トモグラフィーから87000×67000のサイズのデータ行列に遭遇しましたが、非ゼロ要素は0.23%しかありませんでした。
大きな行列は扱いにくいです。そのため、特にデータ行列に多くのゼロが含まれている場合、より小さな行列を使ってデータを要約できないかと考えるのは自然です。これを行うための様々な技術が存在します。例えば、主成分分析や線形判別分析などです。これらの方法の欠点は、元のデータ行列の非負性を保持しないため、結果の解釈が難しくなる可能性があることです。ここで非負値行列因子分解が登場します。
非負値行列因子分解(NMF)は、非負のデータ行列Sを取り、それを背の高い細い行列W(特徴行列として知られる)と短い幅広い行列H(係数行列)の積に因子分解しようとします。
WとHの両方が非負です。これを以下の図に示します。等号ではなく≈が存在することに注意してください。正確なNMFが存在しない可能性があるためです。実際には、NMFアルゴリズムは最適解を得るのではなく、許容可能な解に向けて反復します。
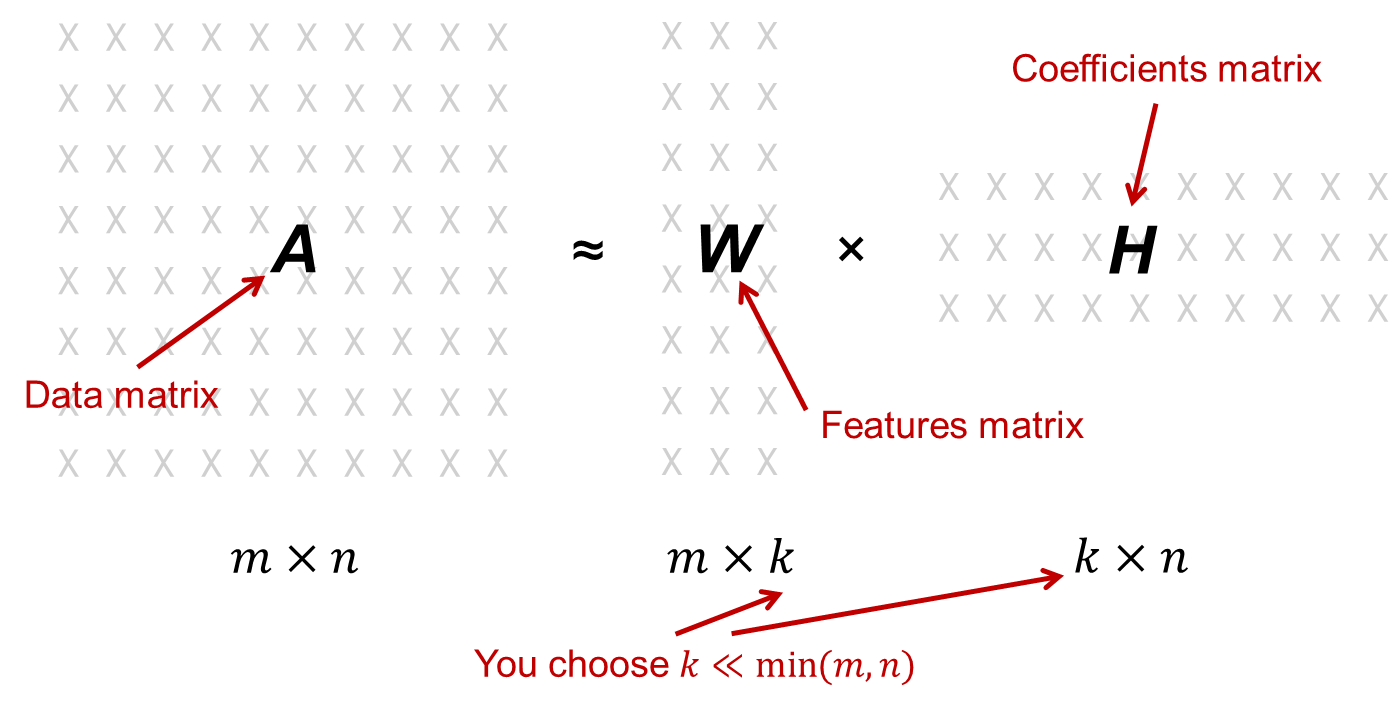
ここで、将来の参照のためにデータ行列をwordcounts.txt
ファイルに出力します。
print("Printing data matrix to file")
= open('wordcounts.txt', 'w')
f2
= ' '*14
st for i in range(n_links):
= 'link ' + str(i+1)
tmp += tmp.ljust(8, ' ')
st
print(st, file=f2)
for i, v in enumerate(words):
= v.ljust(14,' ')
st for j in range(n_links):
+= str(int(a[i,j])).center(8, ' ')
st print(st, file=f2)
f2.close()
データ行列をファイルに出力中
非負値行列因子分解の計算
ここで、非負値行列因子分解を使用して、以下の行列 \(w\) と \(h\) を計算します:
\[a = w@h\]
ここで、\(@\) は行列-行列乗算の標準的なPython演算子です。
Mark 27の時点で、nAGライブラリには非負値行列因子分解を計算するための2つのルーチンがあります:real_nmf と real_nmf_rcomm です。ここでは、k=2 を使用して real_nmf を使用します。
= a.shape[0]
m = a.shape[1]
n = 2
k = 1e-6
errtol = 500
maxit = 5842
seed
= real_nmf(a, k=2, seed=seed, errtol=errtol, maxit=maxit) w, h
C:\ProgramData\Anaconda3\lib\site-packages\ipykernel_launcher.htm:8: NagAlgorithmicWarning: (nAG Python function naginterfaces.base.matop.real_nmf, code 7:7,99992)
** The function has failed to converge after 500 iterations.
** The factorization given by w and h may still be a good enough approximation
** to be useful. Alternatively an improved factorization may be obtained by
** increasing maxit or using different initial choices of w and h.
w.shape
(1825, 2)
h.shape
(2, 15)
因数分解は厳密ではありませんが、有用であるのに十分近いことを期待しています
= norm(a-w@h)/norm(a)
resnorm print("norm of residual:")
print(resnorm)
norm of residual:
0.4996361512168467
NMFの強みは、非負性を保持することで因子WとHの解釈がより容易になることです。一般的に、Wは異なる変数がどのようにkの特徴にグループ化できるかを示し、それらの特徴は何らかの形でデータを表現します。行列Hは、元の観測値がこれらの特徴からどのように構築されているかを示し、非負性によりこれが純粋に加算的な方法で行われることが保証されます。
これを理解する最良の方法は、元の例に戻ることです。15のウェブページに対する1834×15の単語頻度行列があったことを思い出してください。nAGライブラリのルーチンreal_nmfを使用してこれを因子分解し、k=2を選択しました。その結果、1834×2の特徴行列Wと2×15の係数行列Hが得られました。これらについて順番に議論しましょう。
Wの各列は、記事から得られた1834の異なる単語の特定の重み付けグループに対応します。列の要素が大きいほど、対応する単語がより重要とみなされます。Wを全体的に表示するのではなく、各列の上位10個の要素を見ることで、最も重要な単語を確認できます。結果は以下に示されています。
for i in range(k):
= sorted(words, key=lambda x, ind=i: w[words.index(x),ind], reverse=True)
tmp = "\nThe most important words in column " + str(i+1) + " of w are:"
st print(st)
print(tmp[:10])
以下はwの列1における最も重要な単語です: [‘quot’, ‘deal’, ‘brexit’, ‘ssrcss’, ‘class’, ‘delay’, ‘parliament’, ‘minister’, ‘prime’, ‘e1no5rhv0’]
以下はwの列2における最も重要な単語です: [‘quot’, ‘trump’, ‘president’, ‘women’, ‘mccain’, ‘class’, ‘ssrcss’, ‘nielsen’, ‘border’, ‘security’]
これらのリストを見ると、最初の列がトランプに、2番目の列がブレグジットに対応していることに同意していただけると思います。非負値行列因子分解が2つのウェブページのカテゴリを正常に検出したようです。これらを0と1の数字で表しましょう。では、このNMFを使って個々のページを正確に分類できるでしょうか? そのためには係数行列Hを見る必要があります。
表示目的でhをpandasのデータフレームに変換します
# 表示目的のためにhをpandasデータフレームに変換する
import pandas as pd
= pd.DataFrame(
display_h
h,=[
columns"link1","link2","link3","link4","link5","link6","link7","link8","link9",
"link10","link11","link12","link13","link14","link15",
]
)'precision', 3)
pd.set_option( display_h
link1 | link2 | link3 | link4 | link5 | link6 | link7 | link8 | link9 | link10 | link11 | link12 | link13 | link14 | link15 | |
---|---|---|---|---|---|---|---|---|---|---|---|---|---|---|---|
0 | 1.226e+01 | 1.195e-15 | 5.253 | 50.795 | 1.353 | 6.003e+00 | 5.638 | 10.739 | 76.032 | 1.913e+01 | 1.195e-15 | 34.929 | 1.195e-15 | 4.433 | 5.544e+01 |
1 | 9.842e-16 | 5.373e+01 | 5.094 | 3.985 | 3.680 | 9.842e-16 | 7.298 | 24.121 | 0.117 | 9.842e-16 | 4.388e+01 | 0.353 | 2.589e+01 | 1.619 | 9.842e-16 |
この係数行列のサイズは2×15です。各列のエントリは、その特定のウェブページが2つのカテゴリにどの程度適合するかを示しています。各ページは、単に列内の最大のエントリを選択することでカテゴリに割り当てられました。結果は以下の通りです。各リンクの横にある数字は、NMFによってどのようにカテゴリ分けされたかを示しています。カテゴリ分けが正しいかどうかは、あなた自身で判断してください!
for i, link in enumerate(links):
= 0 if h[0,i] > h[1,i] else 1
category = '"' + (titles[i][0]) + '"'
title = 'Article ' + title.ljust(78,' ') + ' is in category ' + str(category) + [" (Brexit)"," (Trump)"][category]
st print(st)
Article "Brexit delay: How is Article 50 extended? - BBC News" is in category 0 (Brexit)
Article "Kirstjen Nielsen: Walking a tightrope working for Trump - BBC News" is in category 1 (Trump)
Article "Trump homes plan at Menie being recommended for approval - BBC News" is in category 0 (Brexit)
Article "Theresa May at her worst during Brexit speech - Mark Drakeford - BBC News" is in category 0 (Brexit)
Article "President Trump shows map of 'IS defeat' - BBC News" is in category 1 (Trump)
Article "Brexit: What happens now? - BBC News" is in category 0 (Brexit)
Article "Trump spooks markets with China trade tariffs warning - BBC News" is in category 1 (Trump)
Article "A tale of two Trumps: Jair Bolsonaro goes to Washington - BBC News" is in category 1 (Trump)
Article "Brexit: Theresa May to formally ask for delay - BBC News" is in category 0 (Brexit)
Article "Corbyn calls for compromise to avoid no-deal Brexit - BBC News" is in category 0 (Brexit)
Article "Trump: I didn't get a thank you for McCain funeral - BBC News" is in category 1 (Trump)
Article "Brexit: EU leaders agree Article 50 delay plan - BBC News" is in category 0 (Brexit)
Article "Trump: Time to recognise Golan Heights as Israeli territory - BBC News" is in category 1 (Trump)
Article "Brexit: MPs urged not to travel home alone as tensions rise - BBC News" is in category 0 (Brexit)
Article "'Cancel Brexit' petition passes 2m signatures on Parliament site - BBC News" is in category 0 (Brexit)